In conventional machine learning, a computer is used to minimize a cost function that specifies a desired task, using global information about the entire network. We have now demonstrated how machine learning tasks can be learned and performed without either a computer or global information by taking advantage of physics via a scheme called coupled learning1. Specifically, twin variable-resistor networks are run under different boundary conditions and corresponding edges are compared against only each other for determining resistance updates2. This permits straightforward hardware implementation (see figure) and offers significant scaling advantages in speed and power-consumption for very large networks. Our scheme can potentially be applied to fluidic and mechanical networks. As such, it holds great promise for creating broad new classes of metamaterials that can learn complex and adaptable functionalities.
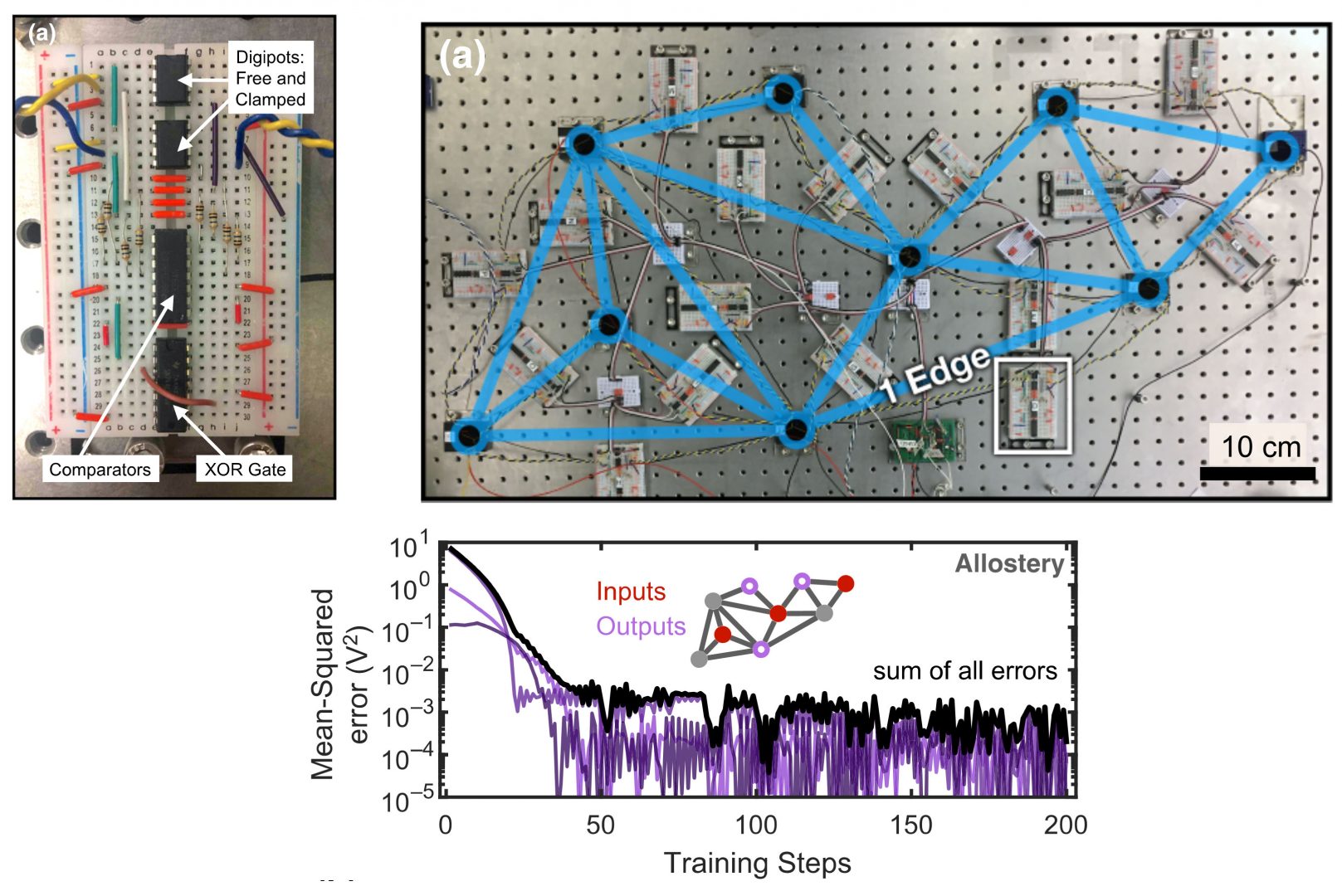
Physics-driven electronic learning machine. (Left) One variable-resistor edge with circuity to adjust its resistance each clock cycle. (Right) A randomly-connected network of fifteen such edges as the repeat unit. (Bottom) The three red nodes are designed as the inputs and the three open nodes are designated as the outputs. During the training phase for an “allostery” task, specific voltages are applied to the inputs, and the edges self-adjust until the output nodes evolve to the desired values. Regression and classification tasks were also demonstrated using appropriate supervision during the training phase.
References:
[1] Stern M, Hexner D, Rocks JW, Liu, AJ. Physical Review X 2021; 11, 021045.https://doi.org/10.1103/PhysRevX.11.021045 [2] Dillavou S, Stern M, Liu AJ, Durian DJ. Physical Review Applied 2022; 18, 014040.
https://doi.org/10.1103/PhysRevResearch.4.L022037